Every CAE professional has wondered: Can AI-driven predictions be as trustworthy as traditional simulations? Typically, Finite Element Analysis (FEA) and Computational Fluid Dynamics (CFD) require substantial computational resources and time. However, AI-powered solutions are now redefining efficiency, using Geometric Deep Learning to deliver faster and more accurate physics predictions.
In a recent deep dive session with Shubhamkar Kulkarni from Altair, we explored how PhysicsAI simplifies simulation workflows, allowing engineers to generate rapid physics predictions without requiring extensive data science expertise. This blog post breaks down the core concepts behind PhysicsAI, its advantages over conventional machine learning models, and its practical applications in engineering and design.
Geometric Deep Learning in PhysicsAI
Traditional machine learning models for CAE often rely on surrogate modeling techniques, where regression-based approaches are used to map input parameters to specific outputs. These models require explicit parameterization, which limits their ability to handle complex geometrical variations.
PhysicsAI, on the other hand, leverages the power of Geometric Deep Learning to process 3D mesh data directly. Instead of relying solely on numerical inputs, it learns patterns from past simulation results and applies them to new designs. This approach allows for:
- Handling non-parametric, organic geometries without predefined input constraints.
- Generating full 3D field predictions rather than just scalar values or curves.
- Accommodating a wider variety of engineering applications, from structural and CFD analysis to electromagnetics and healthcare simulations.
Testing a PhysicsAI Model
So, what are the key differences that make PhysicsAI more accurate and flexible than traditional machine learning models?
Key Differentiators Between Traditional Machine Learning and PhysicsAI
One of the biggest limitations of traditional regression models is their inability to integrate new data seamlessly. If new parameters or geometrical variations emerge, an entirely new surrogate model needs to be trained from scratch. PhysicsAI eliminates this restriction by dynamically incorporating both implicit geometric features and explicit parametric inputs.
Moreover, while traditional models typically yield single-value predictions, PhysicsAI can generate comprehensive 3D field outputs, such as stress distribution, displacement fields, and failure modes. This holistic prediction capability makes it far more versatile for engineering simulations.
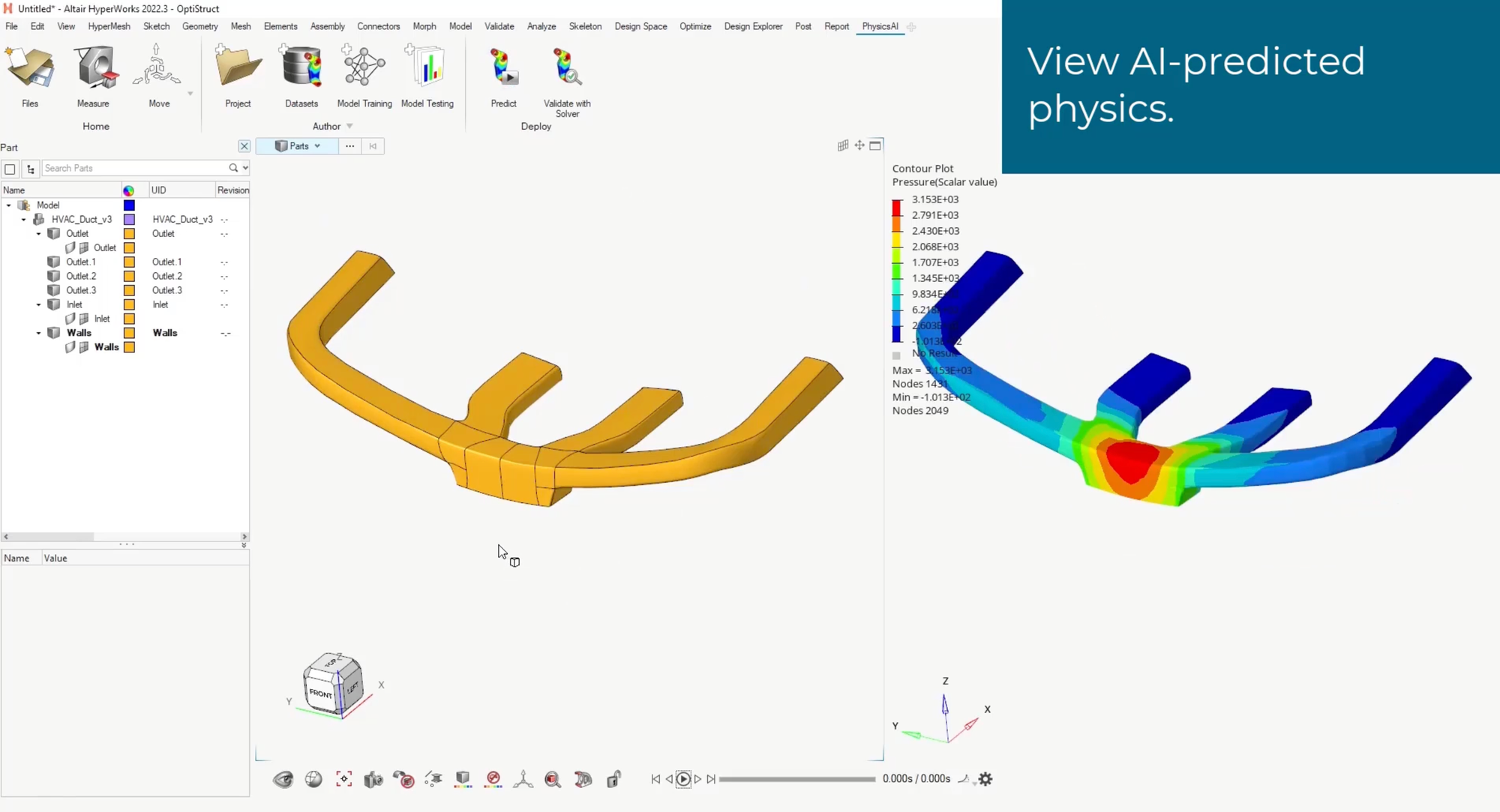
In addition to enhanced prediction capabilities, PhysicsAI streamlines the entire CAE workflow. Traditionally, a designer develops a CAD model and hands it over to a simulation team, where meshing, boundary conditions, and solvers are applied. This process can take hours or even days, significantly slowing down the iterative design cycle.
With PhysicsAI, a pre-trained deep learning model can be shared across teams, enabling designers to generate physics predictions within seconds. Instead of waiting for manual simulation results, engineers can quickly test different design variations, optimizing performance in real-time. These AI models can be deployed locally or via Altair’s cloud platform, further enhancing accessibility and collaboration.
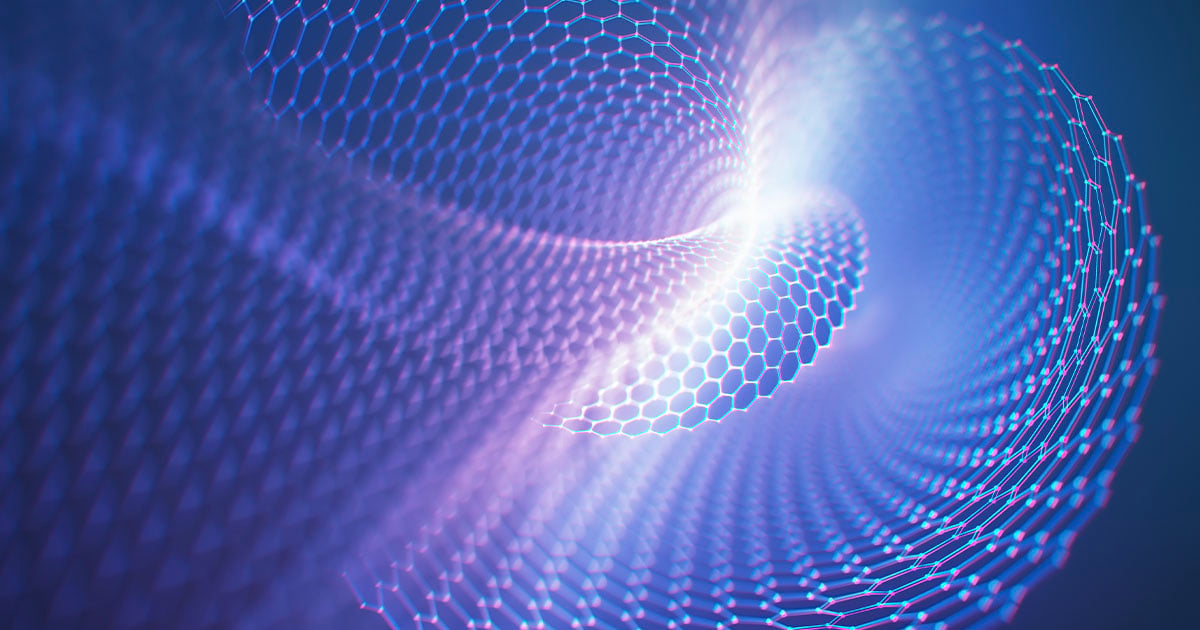
Ensuring Model Accuracy and Trustworthiness
One of the fundamental challenges when integrating AI into simulation-driven workflows is ensuring that predictions are accurate and reliable. Since engineering simulations often guide critical design decisions, AI-generated results must align closely with traditional physics-based methods. To achieve this, PhysicsAI incorporates a multi-layered validation approach.
A crucial step in model validation involves splitting the dataset into training and test sets. By reserving a portion of the dataset for validation, engineers can assess whether the model generalizes well beyond the training data. This ensures that the AI is not merely memorizing patterns but can make accurate predictions on unseen designs.
PhysicsAI also employs a similarity score metric, which evaluates how different a new design is compared to those in the training dataset. This metric indicates confidence in the AI-generated results, helping users gauge the level of trust they can place in a particular prediction. Additional scrutiny or supplemental training data may be needed to enhance model performance if the similarity score is low.
Another key component of model trustworthiness is comparative visualization. Engineers can juxtapose AI predictions with traditional simulation outputs, allowing for direct comparison and validation. By overlaying results, discrepancies can be analyzed, and necessary refinements can be made to improve model accuracy.
By implementing these robust validation strategies, teams can ensure that PhysicsAI models deliver meaningful, accurate, and actionable insights. This systematic approach fosters confidence in AI-assisted simulations and enables engineers to harness AI’s efficiency without compromising on reliability.
Finding the right trade-off between Accuracy and Efficiency in AI-driven Simulation Models
Balancing computational efficiency with prediction accuracy is a crucial challenge in AI-driven CAE. Large-scale models with multiple load cases can be computationally expensive, but PhysicsAI offers several optimization strategies:
- Transfer Learning: Instead of starting from scratch, transfer learning allows engineers to leverage pre-trained AI models and fine-tune them with new data. This significantly reduces computational overhead while improving the model’s ability to generalize to new scenarios. By reusing knowledge from existing simulations, transfer learning accelerates the training process and enhances accuracy without requiring vast amounts of new data.
- Selective Model Scope: Rather than predicting all responses simultaneously, engineers can refine their focus on critical output variables that are most relevant to the simulation objective. By narrowing the scope, the AI model can dedicate its computational resources more effectively, ensuring higher precision in key predictions while reducing overall processing time.
Comparing Head-Impact Predictions
- Mesh and Data Pruning: Large simulation datasets often contain non-essential elements that can inflate computational demands. By carefully filtering input data and excluding less significant features, engineers can optimize model performance without sacrificing accuracy. Strategies such as simplifying complex geometries, eliminating redundant nodes, and focusing on key stress regions help streamline processing and improve prediction efficiency.
These strategies enable engineers to maintain high accuracy while ensuring the AI model remains efficient and practical for real-world applications. By adopting a targeted and strategic approach, organizations can maximize the benefits of AI-driven simulation while minimizing unnecessary computational costs.
Conclusion
The integration of AI in physics-based simulations marks a major advancement in engineering workflows. With Altair’s PhysicsAI, designers and simulation engineers can dramatically reduce the time required for design evaluations while maintaining high accuracy. By leveraging Geometric Deep Learning, this technology allows for more flexible and scalable predictions, overcoming the limitations of traditional surrogate models.
As AI continues to evolve, its role in CAE will expand, driving more intelligent and automated design processes. Adopting solutions like PhysicsAI not only enhances efficiency but also empowers engineers to explore a broader design space with greater confidence.
How to Learn More
For deeper insights into AI-driven CAE models with Altair’s PhysicsAI, watch this in-depth deep dive or visit Altair’s website.