Introduction
Batteries are the backbone of modern technology, powering devices from electric vehicles to smartphones. Their efficiency, safety, and longevity have a profound impact on the industries they serve, making battery modeling and management systems (BMS) essential tools in optimizing performance. By simulating battery behavior, engineers can uncover valuable insights, predict performance under various conditions, and design solutions to overcome critical challenges.
Watch our podcast on Battery Modeling and BMS with Xiangchun Zhang from Mathworks
As technology advances, battery modeling continues to evolve, addressing complex questions about aging, efficiency, and safety. This blog post explores the foundational approaches to modeling, the challenges engineers face, and the trends shaping the future of battery technology.
The Purpose of Battery Modeling
Modeling plays a vital role in understanding and improving batteries. It allows engineers to study how batteries age, simulate their behavior under different conditions, and design solutions to make them safer and more efficient. For example, simulation can reveal why batteries lose capacity over time, offering insights into how to extend their lifespan. Similarly, models are used to design cells and packs that maximize energy density, enabling devices like electric vehicles to travel farther on a single charge.
Beyond design, battery models also support the development of battery management systems. These simulations provide a safe environment to test algorithms that control charging, discharging, and thermal management. In doing so, engineers can refine their designs without risking damage to real-world components, accelerating innovation and reducing costs.
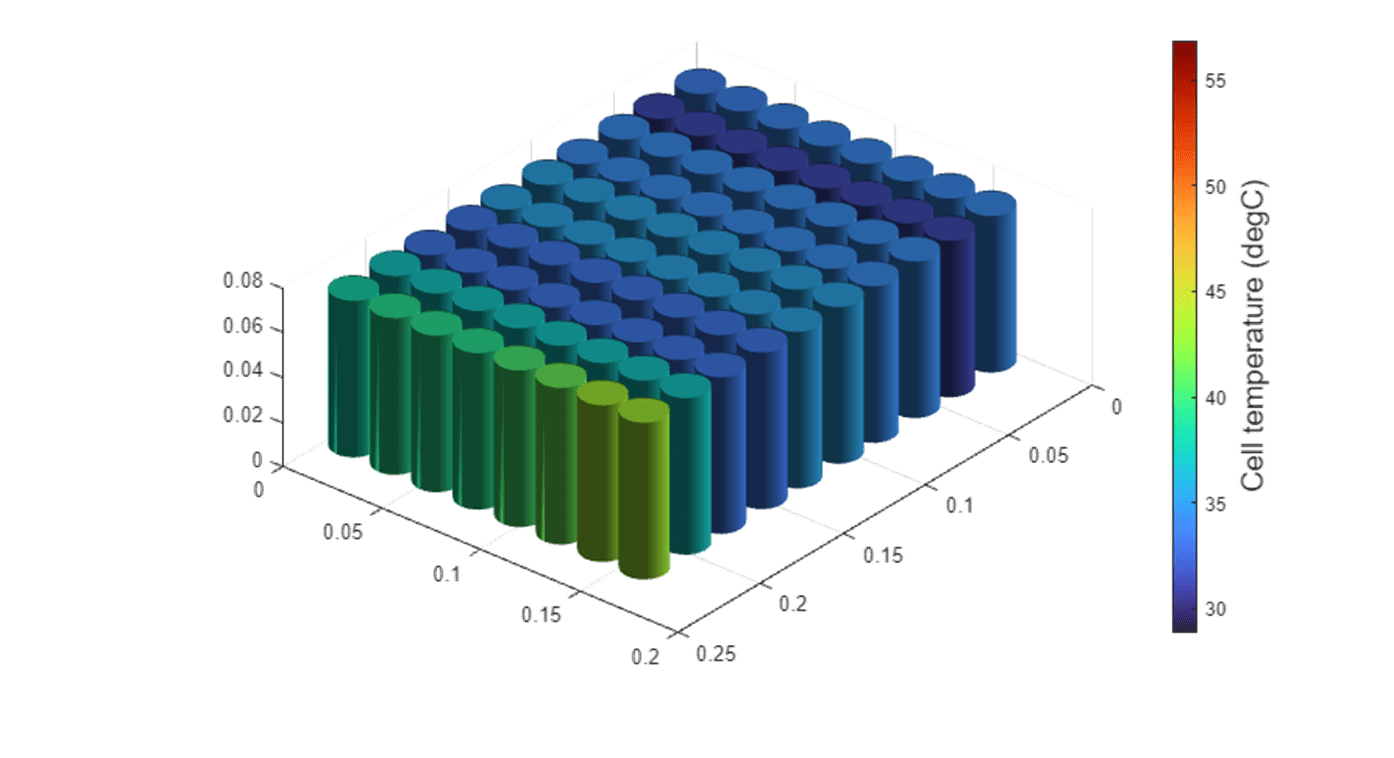
Different Approaches to Battery Modeling
Battery modeling techniques fall into three main categories: electrochemical models, data-driven models, and equivalent circuit models. Each has unique strengths and applications, making it essential to choose the right approach based on the specific use case.
Electrochemical models are grounded in physics, using equations to simulate lithium-ion transport and chemical reactions. They are particularly useful for understanding the internal mechanisms of batteries and predicting how they age. However, their complexity often requires significant computational resources, which can make them less practical for real-time applications.
In contrast, data-driven models rely on patterns found in large datasets. By training these models on experimental or simulation data, engineers can achieve faster predictions without requiring deep knowledge of electrochemistry. These models excel in uncovering trends, such as battery degradation over time, but their accuracy depends heavily on the quality and diversity of the data.
Bridging the gap between these two approaches are equivalent circuit models. These simplified representations use resistors and capacitors to mimic battery behavior, offering a balance between accuracy and computational efficiency. Equivalent circuit models are widely used in system-level simulations, particularly for developing and testing BMS algorithms.
Overcoming Challenges in Battery Simulation
Despite their potential, battery models face significant challenges. The complexity of batteries arises from their multidisciplinary nature, requiring simulations to integrate electrical, thermal, and mechanical behaviors. For example, as batteries charge and discharge, they generate heat, which can affect their performance. Modeling these interactions accurately is crucial for understanding how batteries behave in real-world scenarios, such as during rapid charging or in extreme temperatures.
Another major challenge is predicting how batteries age. Aging occurs through intricate chemical and physical changes, often influenced by factors like temperature and usage patterns. While electrochemical models provide detailed insights, they can be resource-intensive, while data-driven approaches require extensive, high-quality data to capture subtle aging mechanisms. Finding the right balance between these approaches remains an ongoing effort in the field.
Recent advancements are helping engineers address these challenges more effectively. For instance, modern tools now allow for coupling electrical and thermal simulations, enabling a more comprehensive understanding of battery performance. Additionally, advancements in 3D thermal modeling provide detailed insights into temperature distribution across battery cells and packs, a key factor in ensuring safety and efficiency.
Another innovation lies in reduced-order modeling, where high-fidelity simulation data is simplified into faster, more computationally efficient representations. This technique bridges the gap between detailed simulations, like CFD or FEA, and system-level analysis, making it easier to integrate complex battery behavior into broader applications, such as electric vehicles.
Emerging Trends in Battery Technology
The future of battery technology is driven by the need for greater safety, higher energy density, and reduced costs. Solid-state batteries, which replace flammable liquid electrolytes with solid materials, are one promising development. By eliminating some of the risks associated with traditional lithium-ion batteries, solid-state designs offer enhanced safety while also enabling higher energy storage capacity.
Digital twin technology is another exciting trend. These cloud-based virtual models provide real-time monitoring and predictive capabilities, allowing engineers to detect anomalies and optimize battery performance over time. Coupled with advances in BMS algorithms and increased computing power, digital twins could revolutionize battery management by providing more accurate predictions and enabling proactive maintenance.
Conclusion
Battery modeling and management systems are essential to advancing energy storage technology. By leveraging a combination of electrochemical, data-driven, and equivalent circuit approaches, engineers can address complex challenges and unlock new ways for efficiency and safety. With innovations like solid-state batteries and digital twins on the horizon, the future of battery technology promises to be transformative.
As engineers continue to refine these tools, they pave the way for safer, more efficient, and more sustainable energy solutions. Whether optimizing designs or implementing advanced BMS algorithms, battery modeling remains at the forefront of technological progress, fostering a world powered by cleaner and more reliable energy.